SME-Driven Experiments
A SME-driven experiment is the demonstration of the development and implementation of an AI-based solution in a real industrial environment. It is driven by the collaboration among a technical provider who collects the needs and develops the AI solution and an industrial company who explains the current AS-IS scenario and demonstrates the feasibility of the adoption of the solution. The beneficial impact of the use of AI will be evaluated through the monitoring of specific Key Performance Indicators (KPIs).
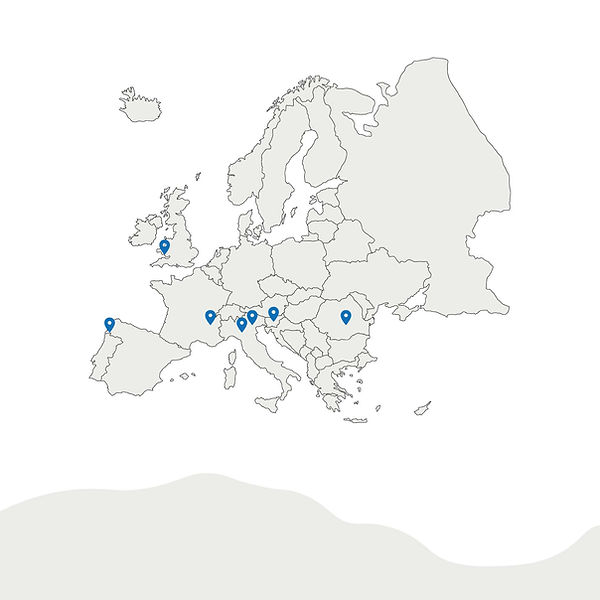
Experiments
Real time monitoring for control and detection of production – Lombardy (IT)
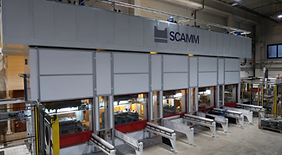
The use case focuses on the application of monitoring technologies for process self-adaptivity to a sheet metal pressing line as any variation in processes’ parameters could lead to non-conformity of the final product. The plant consists of five presses in series that have the task of gradually deforming the sheet metal by applying a predefined load. The line is currently equipped with a control software that allows the setting of process parameters while monitoring is limited to specific technical parameters. The integration of the line control software with edge technologies will allow the data to be accessed and processed, enabling the quantitative characterization of the line’s operating conditions, the assessment of process quality and the determination of any deviations in an objective manner. Moreover, through edge computing technologies, it is possible to apply AI methodologies to operating data to carry out knowledge extraction, allowing to pass from quantitative data to structured information.
AI and Digital Twins for agility in mould making - Auvergne Rhone Alpes (FR)

The Experiment aims to demonstrate the impact of Artificial Intelligence in shopfloor regarding productivity and agility. AI will be used as a decision making tool to organize the manufacturing sequences in a shopfloor where resources are share for different kinds of business unit. It will have to be link to PERNOUD MES system to analyse the current status of every task and predict if some delays (or advance) are expected. In case of delays (or advance), alerts will be launch to inform the users, and some advices to improve the planning will be sent to the users. Advices will be defined to limit the impact according to PERNOUD decision rules. The final call will always be done by the user, a human, with ability to not follow the tools advices.
AI-based autonomous machine for safer faster agriculture – Trentino (IT)

Aim of the experiment is to create an autonomous agricultural machine to make human work more secure. There are in fact some situations where the agricultural land is very steep, and the rows of trees are very narrow. In these conditions the manoeuvre of the machine might be dangerous for the driver and, on some occasions, even fatal. The objective is to create a prototype of a semi autonomous agricultural machine that is able to follow the path between the treelines. To enable this function will be used a pool of sensors robust to the weather condition and the environment, able to recognize road trees, obstacles and measure distances. The hardware will be selected in order to process images and, through AI, evaluate movements depending on the objects around. The system will interface with the existing driving equipment and interact with the machine control unit.
Predictive maintenance and zero-defect production of thermoplastic products - (SI)

The use-case is a small-scale demonstration (for one production cell/process) of predictive maintenance scenario in POLYCOM. The scenario will consist of data infrastructure, edge device and algorithms that will detect and measure the condition of the moulding tools. Demonstrated system will enable operators to continuously monitor the condition of machines and tools and general process operations (changes/events on daily base). Predictive maintenance will be based on active monitoring of the production process, where several data sources will be gathered from the production set-up (material, production parameters and signals, end-quality measurements). Deviations in the performance of specific asset (tool and machine) will be monitored using AI technologies deployed on the local edge device.
AI-enabled Digital Twins for Virtual Commissioning – Galicia (ES)
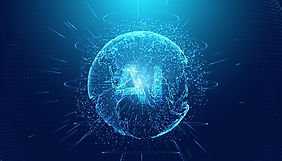.jpg)
For the use-case, digital twin technologies based on the RAMI AAS will be applied as a basis that enables a virtual representation of the plant, focusing not only on process variables obtained from PLCs or MES systems, but also considering virtual carbon footprints and waste related parameters (water and electricity consumption, raw materials waste kg, etc.). This digital twin will be created as a virtual representation at the edge, where models of the different involved machines are continuously created and improved when drifting is detected. Moreover, approaches such as federated and incremental learning algorithms will be used to better create models and extract insights from the process. The main objective is to apply analytic technologies at the edge digital twins to prescribe the best process parameters that minimize waste while keeping quality, efficiency, and productivity at its best. xAI (explainable AI) methodologies will be applied to provide valid feedback to humans regarding the proposed decisions by the algorithms to increase trustworthiness. Security will be considered from the beginning of the use case, thus applying security-by-design.
Intelligent Contextualised Visual System for error reduction – Wales (UK)
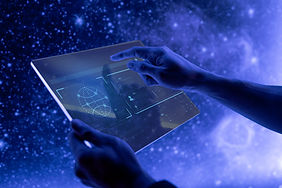
The experiment will integrate different production systems (inc. Industreweb™, PLCs and other control devices), embedded technologies (AI, CPS, IoT) and human operator knowledge, into a single unified digital visualisation platform. Using the AI REDGIO 5.0 architecture, all relevant systems being developed will be connected to create added value within the production process, enabling a variety of outcomes, including the access to specialised knowledge in the production system and to production data for process improvement and efficiency, and the transfer of human-to-machine and machine-to-human knowledge.
Quality Assurance of clothing production - (RO)
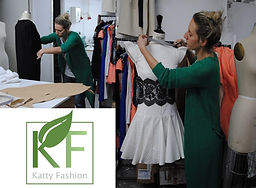.jpg)
The use-case consists in testing the product defect detection system prototype integrating AI-at-the-edge technology developed during the project. System installation with local network connectivity will be set up on the QA production zone of KAF to capture product images with low occlusion and good, consistent lighting. Several relevant types/styles of clothing products will be used for the experiment. The images captured will include examples of both defective and non-defective products to investigate the possibility of defining the non-defective products, by using fuzzy system. Furthermore, the possibility of triggering an automated model building workflow to include a sequence of steps including data augmentation, model training, and postprocessing will be evaluated.